DeepSeek-R1 vs The Human Brain: Lessons in Pattern Recognition and Investment Decision Making
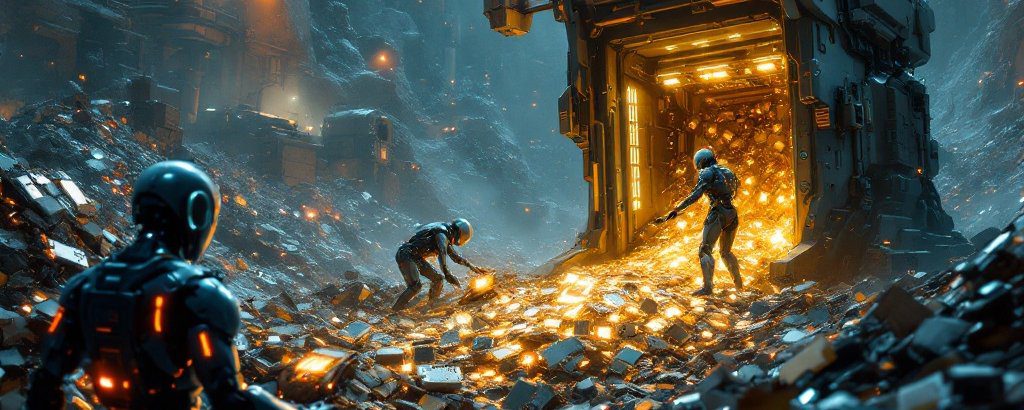
The advancement of AI models like DeepSeek-R1 parallels human cognitive processes, particularly in how we process information and make investment decisions.
Neural Networks – Silicon vs Cells
DeepSeek represents a significant evolution in artificial neural processing, with similarities to how our brains operate through intricate neural networks governed by the C4 Model (Cellular Consciousness Cognitive Control)
DeepSeek’s model architecture mirrors several key aspects of human cognition, including Markov Blankets for information boundaries and the Mixture of Experts approach – similar to how our brain’s specialized regions collaborate.
Energy Conservation – A Double-Edged Sword
In humans, this manifests through Martin Picard’s BEC (Brain Energy Conservation) model, where our mitochondrial networks optimize energy usage. Similarly, DeepSeek’s architecture emphasizes computational efficiency. However, this drive for efficiency comes with a catch – the development of biases as we can see quick default to selecting a known pattern rather than continually engaging in deep analysis and factoring in changing circumstances.Both biological and artificial intelligence systems attempt to prioritize energy efficiency.
Investment Biases: The Pattern Trap
Some common investment biases emerging from our brain’s energy-saving shortcuts include:
- Recency bias: Overweighting recent market performance
- Confirmation bias: Seeking information that confirms existing beliefs
- Anchoring: Fixating on initial price points or valuations
- Herd mentality: Following crowd behavior without proper analysis
These biases often lead investors to rely on pattern recognition rather than conducting thorough bottom-up analysis – a dangerous shortcut in today’s market environment.
The AI Advantage: Systematic Analysis
One powerful application that should be employed when using AI for investment analysis is its ability to generate comprehensive bull and bear cases without emotional attachment. Let’s apply this to current market dynamics: (summary only)
NVIDIA Case Study
- Bull Case: AI infrastructure dominance, high margins, first-mover advantage
- Bear Case: Historical chip industry cyclicality, potential margin compression, valuation risks
- Risk Quantification: Current valuation assumes sustained high margins in an industry historically prone to compression
Historical Context: The Tech Bubble Lesson
Remember the 2000 tech bubble aftermath:
- Amazon’s stock, after a 95% drawdown, took about 15 years to surpass its 2000 high; Microsoft, after a 65% drop, took until the mid-2010s to reach back to its 2000 high; Intel, with an 82% decline, and Cisco, after an 89% drawdown, both still haven’t reached there 2000 peak more than 20 years later.
- Telecom stocks were wiped out; fiber optic component makers, chips, routers, etc., saw margins crushed as data compression related algorithm improvements led to a 99% reduction in bandwidth requirements to send a given file, just as we are now seeing in AI algorithms driving drastic computing efficiency.
- Huge companies once highly coveted like Nortel Networks and Worldcom were driven into bankruptcy as their accounting gimmickry/fraud was exposed. When this bull run ends we will once again see a major change in accounting assumptions leading to huge write downs, massive financial losses and an evaporation of profit margins. (see end note)
- Decade-long recovery periods ensued despite continued growth
- Plus there was no guarantee of eventual recovery to new highs, many stocks crashed 99% on route to oblivion.
Smart Risk Management
The key to my investment success lies in combining:
- Targeting stocks and sectors with a greater than 50%+ confidence levels for significant improvements
- Seeking asymmetric risk-reward ratios
- Focusing on smaller market cap opportunities with 10-20x potential
- Avoiding overvalued large-caps with limited upside
Group Relative Policy Optimization & Market Psychology
Modern AI systems like DeepSeek-R1 employ Group Relative Policy Optimization (GRPO) to learn optimal behaviors within group dynamics. This computational approach parallels how Picard’s Energy Resistance Principle (ERP) explains biological and market behaviors.
Both frameworks reveal something fascinating about resistance patterns – whether in neural networks, cellular systems, or financial markets. The ERP model shows how biological systems resist energy state changes, creating predictable patterns of behavior. Similarly, GRPO demonstrates how AI systems optimize decision-making policies by learning from group interactions.
But here’s the key insight – while DeepSeek-R1 optimizes for computational efficiency, stock markets exhibit additional layers of complexity driven by human emotions and biological energy resistance. This biological-computational parallel becomes particularly relevant during periods of market stress.
Just as cells demonstrate resistance to state changes to conserve energy, market participants resist trend shifts until forced to adapt. This resistance creates predictable patterns – and therefore opportunities – for investors who understand both the biological and computational principles at play.
The crucial difference? While AI systems like DeepSeek-R1 optimize for pure efficiency through GRPO, human market participants operate under the constraints of their biological energy systems. This inefficiency, driven by the ERP model’s resistance patterns, creates the very market dislocations that alert investors can exploit. (Investors that are willing and able to stay current of emerging facts and adjust their investment outlook accordingly)
The Path Forward
Success in today’s market requires:
- Systematic analysis on top of pattern recognition
- Understanding both technological and psychological market drivers
- Maintaining discipline in valuation assessment
- Learning from historical market cycles
Current Market Application
The “Magnificent Seven” stocks demonstrate concerning risk-reward profiles, similar to previous bubble market peaks at cycle tops. While growth potential exists, current valuations suggest limited upside with significant downside risk – a poor risk-reward proposition for value-conscious investors. By understanding how both our brains and advanced AI systems process information, we can better recognize and overcome our cognitive biases. The key is combining technological tools with disciplined analysis while remaining mindful of market history and valuation fundamentals.
I once again expect near term economic downturns to be met with strong stimulus responses and huge infrastructure spending. The bottlenecks that will then develop and are most likely to lead to company margin expansions (and an increase in both investor sentiment and market multiples) will be emerging in the resource sector.
Those that studied resources in the late 90’s and successfully made the transition from tech bull to bear while investing heavily into resources for the 2000-2010 time period experienced not just significant capital preservation but actual massive outperformance and gained financial freedom. Feel free to follow me as I continue to layout the emerging bull and bear cases I see them.
(note: Capital expenditure (capex) on R&D is amortized over its useful life by spreading the cost across the expected total units sold, so if sales plummet due to competition, leading to fewer units sold than anticipated, the per-unit development and production costs rise, necessitating write-offs and revealing previously unrecognized losses, thus correcting the overstated profit. Profits are often hugely overstated because of overly optimistic sales forecasts)